How to Predict Customer LTV Using Mobile Analytics
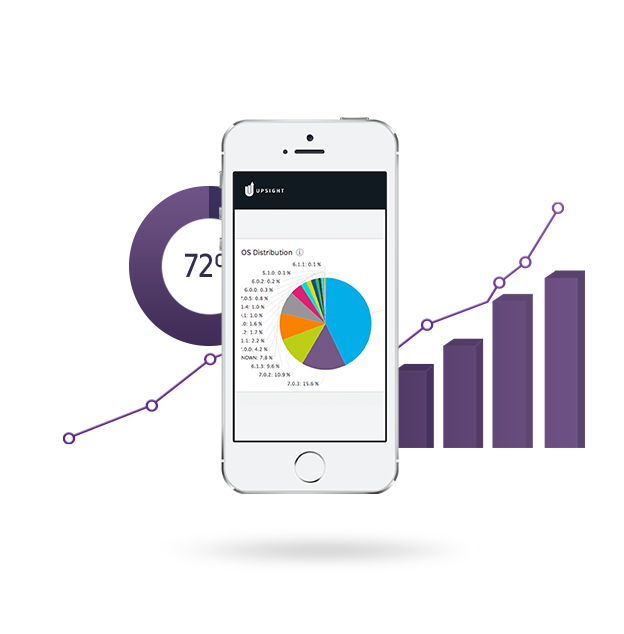
Many advertisers may not yet appreciate the power of mobile analytics. After all, they design ads that fit well on smaller mobile displays, the content and graphics are effective, and the focus groups registered positively. However, analytics can point out significant areas of improvement that can bring salient value to the offering. The relatively low cost often makes an improvement program based on these analytics have an impressive return on investment (ROI) for mobile marketing campaigns.
LTV Evaluation
One example is a common method of measuring LTV by calculating the average value from users over some lengthy period of time, perhaps a year, and watching to see if the average LTV is trending upwards or downwards. The strategy seems solid. All of the key performance indicators (KPIs) form a part of the recorded values. A campaign with an up-trend average LTV may be very satisfied with campaign performance.
However, an alternate analysis may reveal problems. One such strategy uses cohort analysis to report an average revenue per user (ARPU) over the same time period. Many marketers with a trending growth on the average LTV graph may show a plateau behavior on the ARPU cohort analysis graph. Instead of confirming the results of a positive trend, the ARPU actually indicates a plateau and that the positive momentum of customer LTV is petering out. For this reason, the conclusion is exactly the opposite for the alternate methodology: the ship is taking on water and needs attention.
Cohort Analysis
Although the word “cohort” may bring to mind the associates in a bank robbery, in this context, it is a powerful and apropos method of organizing the data. It is an alternate to data sampling, where a group of users presumed to be representative of the entire body are examined by behavior to measure key data. Cohort analysis begins by grouping the users in appropriate groups by behavior before performing the measurement analysis. This has the effect of focusing the results on the behaviors of groups rather than individuals. This can be an important distinction in uncovering performance gaps.
Divergent Results Explained
Many advertisers who have performed both types of analysis on the same campaign and user set are often impressed by the dramatic differences in the results of the two measurement strategies. In one example, an analysis of the marketing campaign for a software provider showed exactly the results described above: an up-trend in average LTV with a plateau in the cohort analysis. By reviewing their cohort identification strategy, they were able to determine that a particular group, those who were slow to upgrade to new product versions, were experiencing superior LTV to those that were early adopters. This in turn led the company to find critical design problems in their latest releases. These problems, revealed by cohort analysis, were masked in the standard average LTV analysis.
True Optimization
By using the proper metrics and analysis techniques, marketers can optimize their campaigns. The proper analyses show which marketing channels produce the greatest results. This allows campaign managers to focus resources on the most profitable, highest-return marketing channels and strategies.