From Pixels to Precision: How Data Labeling Fuels the Brains of Self-Driving Cars
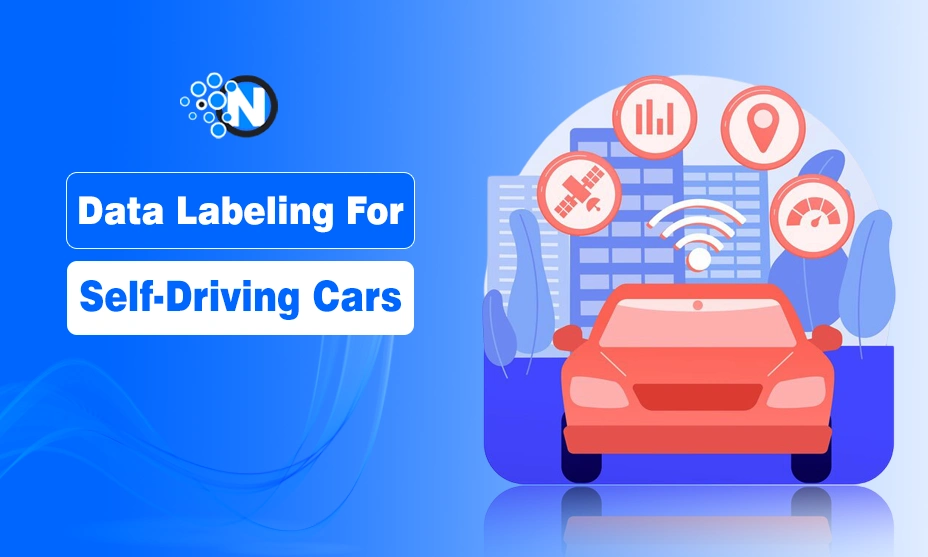
Data labelling is one of the most important but frequently disregarded components driving autonomous vehicles (AVs) from a future idea to a reality. Actually, the foundation of self-driving technology is the apparently basic chore of spotting and marking objects in photos and sensor data.
It links the artificial intelligence (AI) systems producing real-time driving decisions with raw sensor input—pixels, points, and waves. Data labelling is essentially the means by which machines learn to see, understand, and respond to their environment.
In this blog post, I will explain how data labeling transforms raw data into actionable insights that fuel the “brains” of self-driving cars.
Let’s start with understanding data labeling!
What Is Data Labeling?
Data labelling is the practice of marking or annotating raw data like images, text, or sensor outputs to emphasise elements of interest. Data labelling in the context of self-driving automobiles is designating items including vehicles, people, traffic lights, lane markers, and road signs, inside pictures or video frames. Usually human annotators draw bounding boxes, segment images into classes, or give attributes to various parts of a scene, and handle this procedure.
Role of Data Labeling in Autonomous Driving
Autonomous vehicles rely on a complex network of sensors including cameras, LiDAR, radar, and GPS. As the car negotiates its surroundings, these sensors continually compile enormous volumes of raw data. Still, an artificial intelligence system finds this data on its own useless.
Data labeling is the process of marking this raw input, highlighting and tagging people, lane lines, road signs, and other vehicles, so that machine learning algorithms might be trained to recognise and respond to them.
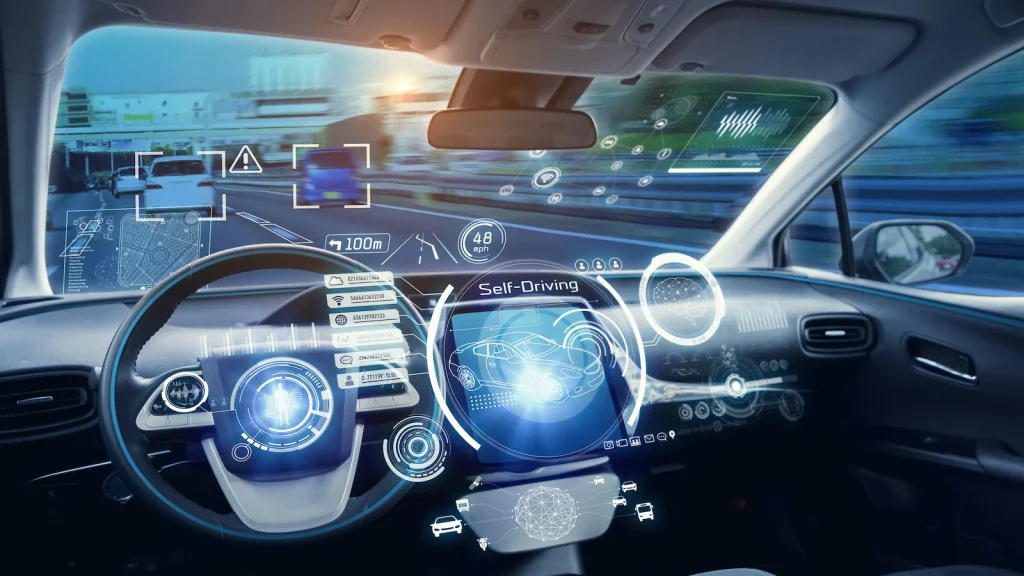
There are multiple types of annotations used, such as:
- Bounding boxes around cars, pedestrians, and obstacles.
- Semantic segmentation that labels every pixel of an image.
- 3D point cloud annotation for LiDAR data to understand object dimensions and distances.
- Temporal labeling to track objects across video frames for motion prediction.
Each of these techniques plays a critical role in teaching AVs how to “see” the road in both simple and complex driving environments.
Challenges in Labeling Data for Self-Driving Cars
Labeling data for autonomous vehicles is not only labor-intensive, but it is also fraught with challenges. First and foremost, the sheer volume of data is staggering. A single AV can generate terabytes of data per day. Ensuring high-quality annotations across this dataset requires a massive and well-trained workforce, sophisticated tools, and robust quality control processes.
Then there’s the problem of edge cases. These are rare or unexpected scenarios, like a pedestrian in a costume or a fallen tree on the road, that the AI must learn to handle. Because such events are uncommon, labeled examples are scarce, yet critical. Labeling edge cases requires extreme attention to detail and often domain-specific expertise.
Moreover, sensor fusion—combining inputs from various sensors—requires synchronized labeling across multiple data types, adding to the complexity. Annotators must understand the spatial and temporal relationships between camera feeds, LiDAR point clouds, and radar data.
Automation and AI-Assisted Labeling: The Future of Annotation
To scale data labeling while maintaining accuracy, companies are increasingly turning to automation and AI-assisted tools. Pre-labeling using algorithms, followed by human review, speeds up the process and reduces manual effort. Advanced platforms use machine learning models to provide initial annotations, which are then refined by human annotators.
Additionally, synthetic data—computer-generated environments and scenarios—offers a promising alternative. By simulating rare edge cases or hazardous situations, synthetic datasets provide a wealth of labeled examples without the cost and risk associated with real-world data collection.
Despite these innovations, human-in-the-loop systems are essential. The nuances of context, motion, and intent (e.g., distinguishing a pedestrian waiting to cross from one simply standing) are still best understood and verified by humans.
Why Quality Matters: The Link Between Labeling and Safety
Data labeling isn’t just about technical accuracy; it’s directly linked to safety. Mislabeling a cyclist as a stationary object or failing to detect a stop sign can have severe consequences. The higher the quality of the labeled data, the better the machine learning models perform in real-world scenarios.
Accurate labelled data helps the systems to become very good in identifying and classifying things. This is especially important in situations when the vehicle has to discriminate between several objects, such a fixed object vs a dynamic one like a pedestrian.
That’s why leading AV companies invest heavily in multi-stage quality assurance, including:
- Cross-validation by multiple annotators
- Automated checks for consistency
- Continuous feedback loops between labeling teams and model developers
Ultimately, the reliability of autonomous vehicles hinges on the precision of their training data. This is where data labeling transforms from a background task into a mission-critical component.
To Sum Up
From lane markings to pedestrian movement prediction, every smart action an autonomous car takes is based on how well its artificial intelligence perceives the environment, a skill it learns from labelled data. Demand for excellent, scalable, context-aware data labelling will grow as the sector runs towards complete autonomy. Although synthetic data and automation present exciting directions, the human element is still crucial in conveying the delicate complexity of actual driving.
Data labelling is the lens through which autonomous cars learn to negotiate with accuracy, safety, and intelligence, far more than just a preparation phase. And it has never been more important, as we hand over the steering wheel to robots to ensure the lens is crystal clear.