Automated Metadata Management: Your Key to Data Organization Success
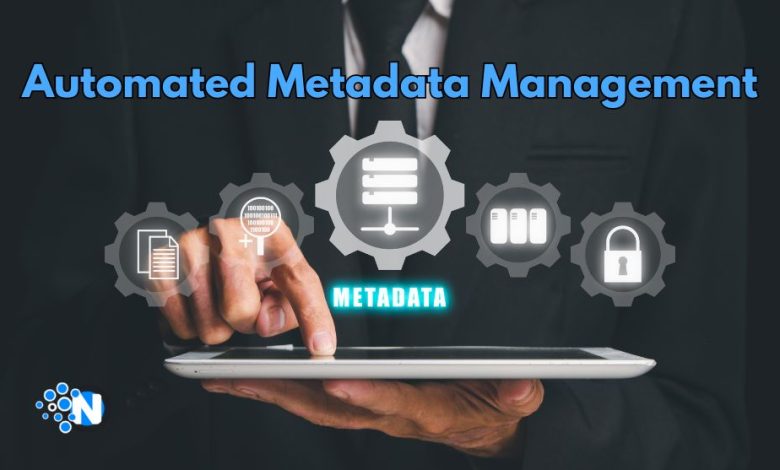
Today’s organizations live and die based on how they leverage the power of data to gain valuable insights that drive competitive advantage. However, with data volumes growing at exponential rates, most find themselves literally drowning in data. The result? They’re unable to leverage it for analytics, reporting, and decision-making efficiently. The root of this problem lies in poor data management.
With improperly managed data assets, it becomes next to impossible to create that coveted ‘single source of truth,’ leading to poor data quality and decision-making. A study even noted that poor data quality costs organizations USD$ 12.9 million every year.
This is where automated metadata management comes in. It has emerged as a foundational practice for any enterprise pursuing data organization maturity. This article will explore this approach, including its benefits for your organization and what the best practices for integration are.
What Exactly is Metadata Management?
Metadata management refers to the administration of ‘data about data,’ encompassing the processes and governance around capturing, organizing, and utilizing metadata to optimize the planning, architecture, security, use, and analysis of data within an organization.
Metadata itself is essentially descriptive documentation that enumerates the structure, meaning, relationships, lineage, and provenance of corporate data assets. Effective metadata management thus unlocks a multidimensional understanding of organizational data ecosystems that drive accessibility, trust, and interoperability.
Benefits of Automated Metadata Management
Here are metadata management benefits that can help optimize your organization’s data ecosystems:
Discoverability and Understandability
Comprehensive metadata facilitates discoverability and self-service understanding of data assets. Auto-generated technical, operational, and business metadata provides a cheat sheet on what datasets contain, eliminating dependence on tribal knowledge. Descriptive tags and content indexing then make these data assets visible and findable via search engines for various analytics use cases.
Relationship mapping highlights linkages between datasets, fostering the identification of additional relevant data sources. Data flow documentation further bolsters understandability by enabling impact analysis when upstream sources change.
Standard business glossaries can also be embedded into metadata dictionaries for consistent interpretation across teams. The combination of technical visibility, content summaries, relationship mapping, and common vocabulary makes the obscurity of data a thing of the past.
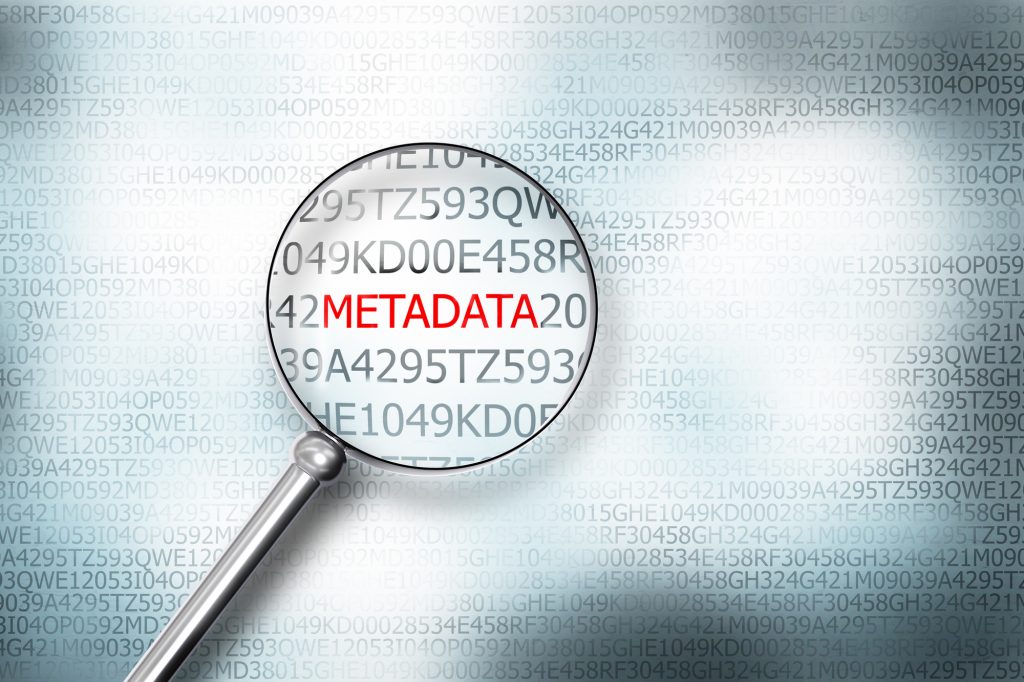
Consistent Governance and Standards
Rules-based automation ensures the consistent application of security, privacy, and data governance controls across cataloged data assets. Embedding data policy triggers within the metadata repository allows violation alerts to be pushed to relevant owners for remediation. Compliance with regulatory mandates can thus be systematically enforced enterprise-wide.
Monitoring metadata also reveals statistics and improvement opportunities around historical governance execution. Tagging datasets with standard definitions, valid value sets, formatting rules, and slack thresholds promotes discipline and upholds baseline data quality across analytical data pipelines.
Power for Integration and Interoperability
Cataloging the intricate relationships between data assets including technical interfaces and semantic linkages powers complex, large-scale data integration initiatives. Matching structural metadata patterns across datasets smooths the combining of big data pipelines and architectures.
Propagating one unified metadata model across new data feeds that enter the organization accelerates its usability by instantly connecting them to existing frameworks. This approach means interfaces and connections need to be mapped only once at the metadata level instead of configured separately for every data integration. Understanding upstream dependencies also allows precise impact analysis to ripple through downstream data systems when changes occur.
Automation of DataOps Processes
Automated crawling of volumes and infrastructure provides continuous auto-profiling, bringing new blindspot datasets into compliance by auto-tagging with appropriate metadata. Application programming interface (API) programs further enhance automation by allowing existing DataOps scripts to leverage rich metadata for orchestrating data pipeline transitions, preparation steps, validation checks, and more.
Embedding data quality rules directly into metadata repositories similarly enables real-time monitoring and threshold alerts. Auto-documentation of data supply chains and lineage via metadata also empowers one-click impact analysis. The reuse of best practice metadata recipes makes it easier to replicate data transformation successes.
Best Practices for Implementation
Metadata management implementation is seen across numerous organizations, and its market value is proof of this. In 2022, this industry was valued at USD$ 1.86 billion, which was a 19.5% growth from previous figures. Businesses that were successful in implementing this solution did reap the dividends.
However, all this metadata magic doesn’t just happen automatically. For organizations struggling with data disorganization, here are five best practices to ensure your metadata management initiatives deliver maximum impact:
- Take stock of your existing metadata
The first step is to identify existing sources of metadata already embedded in various data storages and even individual files. This is key to preventing reinvention of the wheel. It also provides a baseline understanding of the current state of data landscape organization maturity.
- Establish a central metadata repository
Construct an architectural foundation to consolidate metadata from all these distributed sources into a central catalog or repository. This registry acts as the single source of truth to organize your enterprise data assets.
- Automate metadata collection
For maximum completeness and accuracy, continuously collect metadata automatically from source systems using automated crawlers, APIs, or integration connectors. This includes technical metadata like schema definitions, operational metadata like job logs and lineage, and business metadata like data domains and ownership info. Embedding robust auto-profiling capabilities is also key to enabling the discovery of previously unknown datasets.
- Focus on searchability and accessibility
Curating all this metadata is only half the battle, it must be made easily findable, accessible, and understandable to both humans and machines in order to fulfill its value potential. Prioritizing user-friendly but powerful search and API access mechanisms to metadata is crucial for usability. Dictionary-driven business glossaries and data catalogs enhance understanding.
- Trigger actions and embed intelligence
Finally, activate metadata to work for you by using it to drive critical actions. Configure policies, rules, recommendations, and triggers based on metadata to enable processes like data protection, lifecycle transitions, quality alerts, and more. Pushing metadata into data prep, analytics, and ML tools also embeds intelligence that improves their output.
By following these best practices around centralization, automation, accessibility, and activation, organizations can implement metadata management in a way that durably tackles the root causes of data disorganization.
Conclusion
With 3.5 quintillion bytes of data generated every day, data landscapes are getting more complex. As a result, organizations can no longer rely on tribal knowledge and manual approaches to understand their data assets. Fortunately, metadata emerges as the essential ingredient for coherence and optimization in modern data estates.
But to scale across ballooning enterprise data volumes, human-powered tactics must give way to comprehensive automation. This holds the key that unlocks true data accessibility, compliance, and flexibility. With this approach, analytics practitioners are freed from the shackles of obscurity and doubt. The outcome is accelerated delivery of data value to the business—fulfilling the core mandate of any data science team.